Agentic AI
The Next Frontier
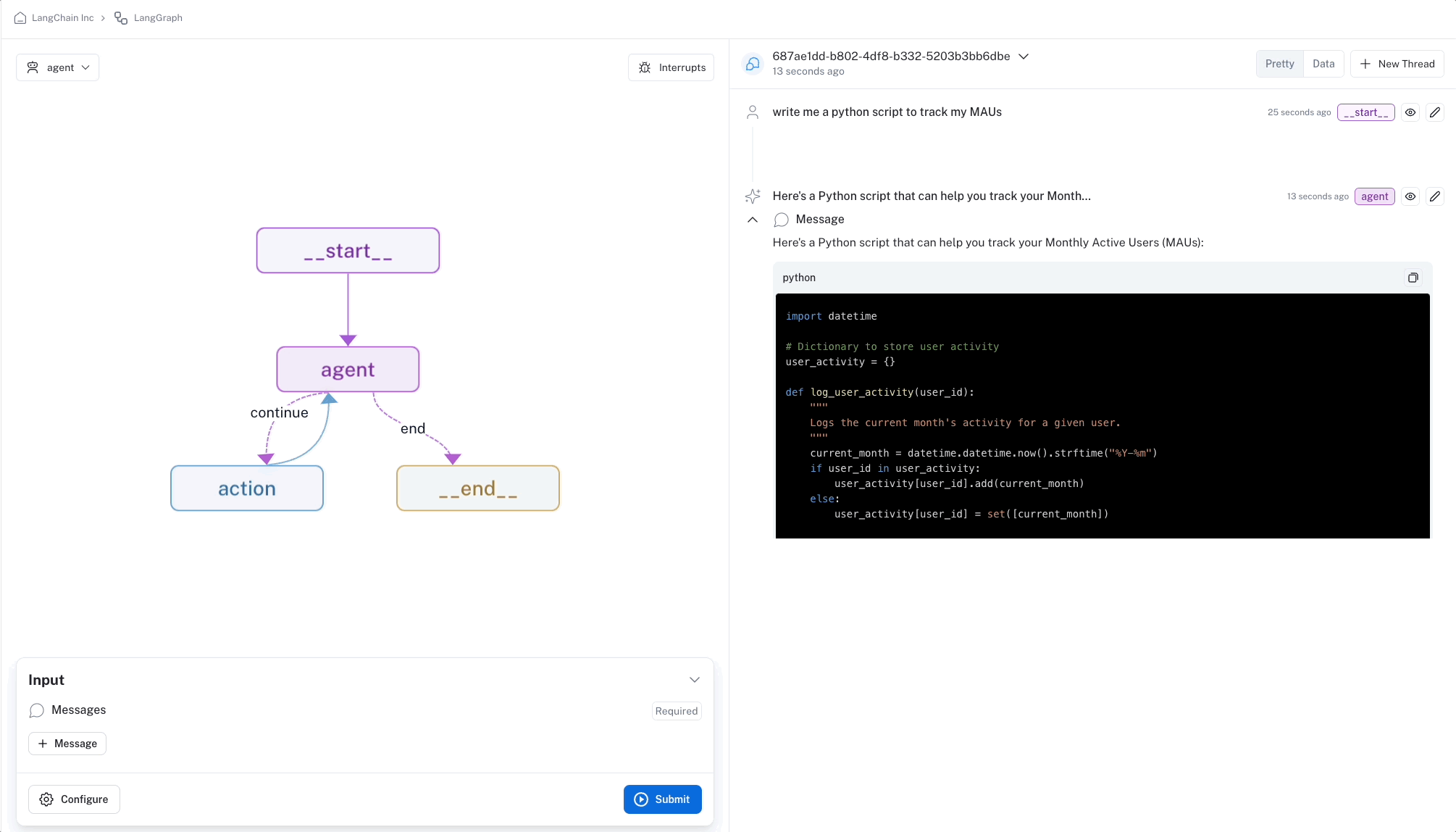
Rumor has it that our AI assistants are becoming more empowered. They’re evolving from simple question-answerers to active agents, capable of performing tasks on our behalf. This shift, long anticipated, is poised to revolutionize our systems.
Large Language Models (LLMs) are morphing into Large Action Models (LAMs), with endless consumer applications from travel bookings to social media management. In the business world, the impact will be even more profound. Ed Agents can create personalized courses, from gathering input to publishing them and tracking engagement. Recruiter AI Agents can streamline the hiring process, from sourcing candidates to scheduling interviews.
This just the tip of the iceberg. Mayfield is pioneering a shift from software as a service (SaaS) to services by software. They have developed a range of AAI agents with human-like features, resembling Pixar characters. These agents cover various roles, including data engineering, sales, cybersecurity, and finance. These agents will soon feel like employees, requiring training, onboarding, and coaching. As they mature, they’ll integrate with more systems, people, and data.
What is Agentic AI?
Agentic AI is a cutting-edge form of AI that empowers autonomous agents to think, act, and learn like humans. By combining techniques like LLMs, machine learning, and reinforcement learning, these agents can analyze data, set goals, and take action independently. This revolutionizes industries and redefines how we interact with technology.
Agentic AI vs. Generative AI
Agentic AI and generative AI (GenAI) are distinct branches of AI, each excelling in different areas. GenAI creates content, while agentic AI takes action. GenAI generates new content, such as text, images, or code. It’s great for brainstorming and creative tasks but relies on human input for context. Agentic AI is autonomous, making decisions and taking actions independently. It can analyze situations, set goals, and learn from experience.
Agentic AI’s Evolution
Early chatbots laid the foundation for Agentic AI. While limited, they showed the potential for AI to interact with humans. LLMs like GPT-3 and GPT-4 transformed AI, enabling natural language understanding and reasoning. This allowed Agentic AI to interact more naturally and make decisions. Machine Learning advancements, like reinforcement learning, have improved Agentic AI’s decision-making skills.
Technological Innovations have fueled Agentic AI’s growth. Enterprise system integration, cloud computing, and AI automation platforms have made it easier to create and manage intelligent agents.
Building Blocks of Agentic AI
Agentic AI is a complex system composed of several interconnected components. These building blocks work together to enable autonomous agents to perceive their environment, make decisions, and take actions.
Key Components:
Perception: This involves the ability of the agent to sense and interpret its environment. This might include visual perception, auditory perception, or other sensory inputs.
Cognition: This refers to the mental processes that allow the agent to think, reason, and learn. Cognitive components might include:
1. Representation: The ability to represent the world in a structured way, such as using symbols or mental models.
2. Reasoning: The ability to draw conclusions from information and make decisions.
3. Learning: The ability to acquire new knowledge and skills through experience.
Action: This is the ability of the agent to interact with its environment, such as moving, manipulating objects, or communicating.
Motivation: This refers to the internal drives or goals that guide the agent’s behavior. It might include seeking rewards, avoiding punishment, or achieving specific objectives.
How They Work Together
Perception-Cognition Loop: The agent perceives its environment, processes the information, and uses it to update its internal representation of the world.
Cognition-Action Loop: The agent uses its cognitive processes to make decisions and plan actions. It then executes these actions in the real world.
Motivation-Action Loop: The agent’s motivation influences its decision-making and actions. For example, a goal-oriented agent might take actions that are expected to lead to achieving its goal.
Building a Functional Agentic AI System
Agentic AI requires a systematic approach to connect its components effectively. Here’s a simplified breakdown:
Data Collection and Preprocessing: Gather and clean data from various sources.
Perception and Feature Extraction: Use computer vision and NLP to extract meaningful features from sensory data.
Goal Representation and Planning: Define clear goals and create effective plans using algorithms like A* search.
Decision-Making: Evaluate options and choose the best action based on goals and current situation.
Action Execution: Control actuators to execute chosen actions.
Learning and Adaptation: Continuously improve the system through various learning techniques.
The implications of Agentic AI are profound, multifaceted, and rapidly evolving. Organizations across all industries must proactively adapt to its transformative potential. While Agentic AI technology is still in its developmental stages, it presents significant challenges as it matures. Central to its functioning are Large Language Models (LLMs), which remain susceptible to hallucinations.
For instance, an agent tasked with retrieving specific web links might inadvertently return incorrect results. This could lead to the LLM becoming trapped in an infinite loop, consuming excessive computational resources and incurring substantial costs for the agent’s human operator. However, the allure of Agentic AI has spurred a surge of developer interest and experimentation. Through iterative refinement and intelligent design, engineers are actively working to mitigate these challenges and create robust, reliable systems.
Three prominent agent frameworks have gained significant traction: LanGraph, AutoGen, and CrewAI.
AutoGen
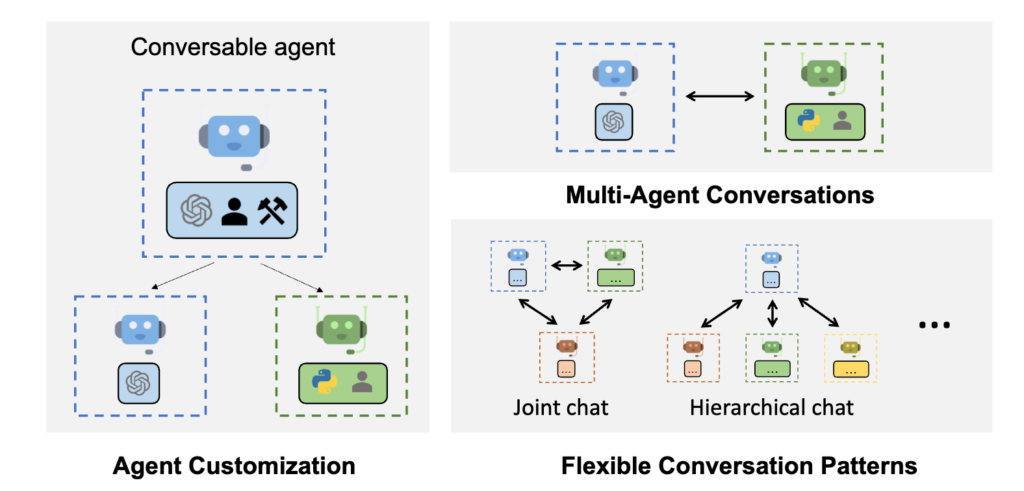
AutoGen, a Microsoft-developed framework, offers a high-level abstraction for creating multi-agent conversational applications. This open-source library empowers developers to construct LLM workflows that involve multiple agents collaborating and tailoring responses to individual users.
Use Case: An enterprise knowledge management system could leverage AutoGen to implement a conversational interface. This system would employ a knowledge base agent, retrieval agent, and dialogue agent to provide users with relevant information and engage in meaningful interactions.
CrewAI
CrewAI, an open-source framework built on LangChain, simplifies the creation and management of collaborative AI agents. Developers can assemble cohorts of specialized agents to tackle complex tasks together.
Use Case: A marketing team might utilize CrewAI to create a series of agents. One agent could gather customer data from social media, another could analyze sentiment, and a third could generate targeted marketing campaigns based on these insights.
LangGraph
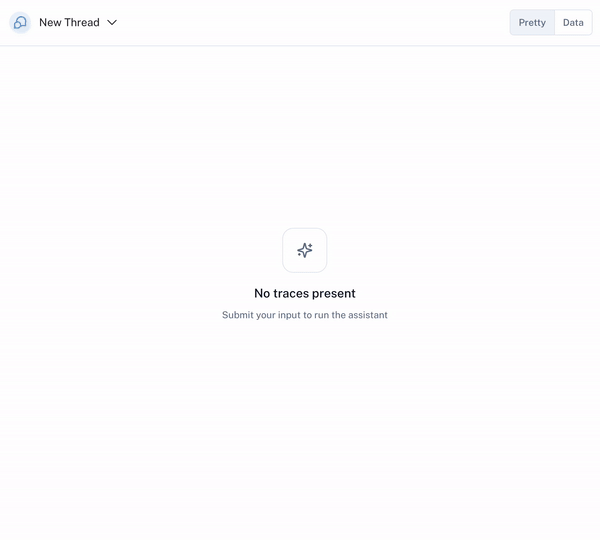
LangGraph, another open-source framework built on LangChain, facilitates the representation of multiple agents within a graph network. This ensures seamless integration and collaboration among agents.
Use Case: An AI research assistant could be constructed using LangGraph. This assistant would include a research content web scraping agent, a processing agent that identifies relevant content and synthesizes curated information, and a generating agent that drafts initial research papers aligned with user goals and objectives.
Organizations across various industries, from content marketing to software development and threat detection, have embraced these AI-powered solutions. A Google Cloud study reveals that 70% of companies have realized a return on investment (ROI) from at least one specific application. A recent McKinsey report estimates that AI technologies will contribute between $2.6 trillion and $4.4 trillion in value to businesses, while simultaneously reducing the overall workload of employees by 50% to 70%.
Agentic AI: Transforming Industries
Sales: Agentic AI automates lead management, allowing tools like Conversica and Relevance AI to engage leads, qualify them, and nurture prospects.
Marketing: Tools like Netcore’s Co-Marketer AI and Salesforce’s Agentforce personalize customer interactions by delivering dynamic, personalized content based on real-time data.
Cybersecurity: Agentic AI-powered tools like Darktrace and Vectra AI monitor network traffic, identify threats, and autonomously initiate responses, ensuring real-time defense against cyberattacks.
Infrastructure and IT Operations: Platforms like Qovery automate IT operations, allowing AI agents to perform tasks like setting up environments, managing scaling, and ensuring uptime. Qovery claims its platform eliminates DevOps hiring needs.
Autonomous Vehicles with Agentic AI
A Personalized Driving Experience!
Autonomous vehicles have become a reality, but with Agentic AI added to mix, your car becomes more than just a mode of transportation. It becomes a personalized driving companion. This vision is becoming a reality thanks to the integration of Agentic AI into autonomous vehicles.
Route Optimization: The car would analyze your past driving patterns, taking into account factors like traffic congestion, weather conditions, and your preferred route. It could even learn your shortcuts and avoid areas you dislike.
Personalized Preferences: The vehicle would remember your preferred temperature, seat position, and music choices, as well as your favorite podcasts and news topics. It could also learn your driving style, adjusting acceleration and braking to match your preferences.
Adaptive Learning: Over time, the car would become more aware of your habits and preferences. For example, if you often stop at a particular coffee shop, it might suggest a detour or recommend a new place based on your past preferences.
Safety Features: Agentic AI could enhance safety features by analyzing real-time traffic data, identifying potential hazards, and taking proactive measures to avoid accidents.
Conclusion
Agentic AI, a sophisticated form of artificial intelligence, is poised to revolutionize industries and redefine how we interact with technology. By empowering autonomous agents to think, act, and learn like humans, Agentic AI offers immense potential for improving efficiency, productivity, and decision-making.